Is the RTX 2070 Super enough for running local AI software, and moreover, is it any good for tasks like gaming and video editing in the current year? Using it in one of my rigs I’m more than qualified to answer this question. Here is how it is.
This web portal is reader-supported, and is a part of the Amazon Services LLC Associates Program, AliExpress Partner Program, and the eBay Partner Network. When you buy using links on our site, we may earn an affiliate commission!
NVIDIA RTX 2070 Super Overall Performance
I’m going to keep this short and down to earth. On my main rig with a pretty dated Intel i9-9900k CPU, 32GB of RAM and the RTX 2070 Super, particularity this exact 3-fan model, I have no problems with:
- Gaming in 2k with high/ultra settings locking my in-game FPS to 60-90 FPS in most games from last years.
- Gaming in 1080p with the exact same settings easily reaching over 120 FPS in many games
- Working with 2k and 4k footage for my YouTube channel, and comfortably editing long-format videos.
- Rendering some relatively complex scenes in Blender using Cycles.
- Running 3 monitors, 1x 2k and 2x 1080p resolution.
There are places in which I found that the card reaches its limits and this is the case especially when it comes to running some games in higher frame rates on high/ultra settings (everything above 60-90fps in 2k resolution is kind of a hit or miss most of the time), and AI software which requires more than 8GB of video memory.
Yes, despite great performance, comparable to the base RTX 3060 when it comes to performance, the 2070 Super does lack in the VRAM department, coming only in 8GB variants which can be a real pain, especially if you want to experiment with more demanding AI software, for instance running larger LLMs locally on your PC.
Stable Diffusion Automatic1111 & ComfyUI
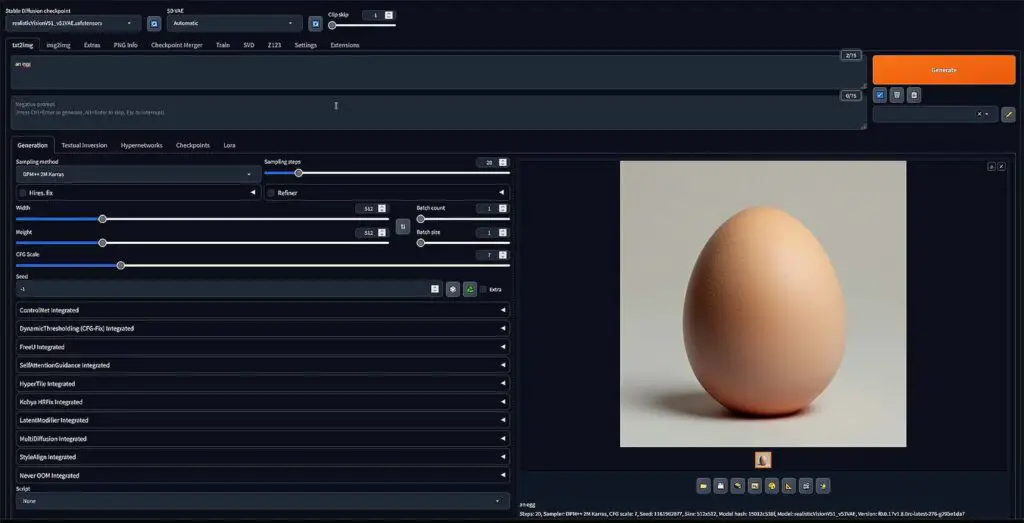
Having spent long hours on playing around with various models, including SDXL, using both the Automatic1111 WebUI and ComfyUI, I can safely say that the 2070 Super is able to generate images blazing fast with the right settings, however it does start to struggle with SDXL models which do require more VRAM to run without offloading the model data to main system RAM and slowing things down a lot. I was able to run SDXL models without a refiner on this card, however it does require some additional tinkering.
The bottomline is: in my personal experience, generating images using the base Stable Diffusion models was a breeze in most setting configurations, training LoRA models for models based on SD 1.5 and 2 was also not only possible but quite efficient, however using SDXL based models, and using them with a refiner without swapping models in VRAM mid-generation has proved to be impossible because of the 8GB video memory limit of this GPU.
Local LLMs and the OobaBooga WebUI
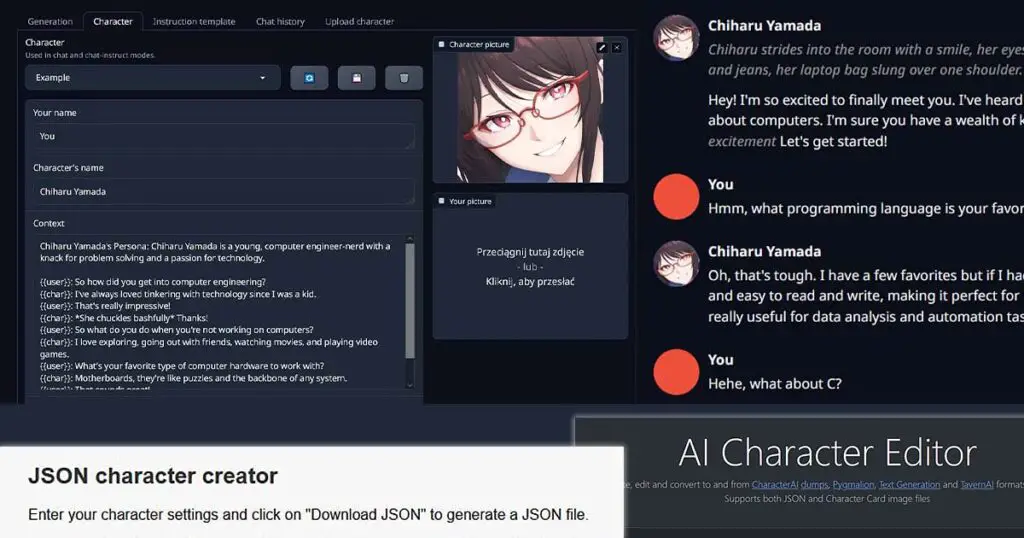
If you’ve already read my guide on the best graphics cards for local LLMs, you probably know that you can never have enough VRAM for running large language models locally. And here comes the sad part. As the RTX 2070 Super doesn’t come with more than 8GB of video memory in any configuration, it’s simply not a good pick if you want to
Sure, you can run smaller, 7B open-source LLM models using the OobaBooga WebUI using a card like this, and in fact, I’ve done so when making my main tutorial for this cool piece of software. However, in these less than ideal conditions you won’t be able to load up any higher quality models with more parameters, and even have any longer conversations with your AI assistant because of the low context window settings forced by the VRAM limits. That’s just how it is.
Refer to the article I mentioned above if you want to know which GPUs do feature 12, 16 and 24GB of VRAM and are more than enough for playing around with local large language models. There are some budget options there too!
Live Voice Changing, Voice Cloning & AI Vocal Covers
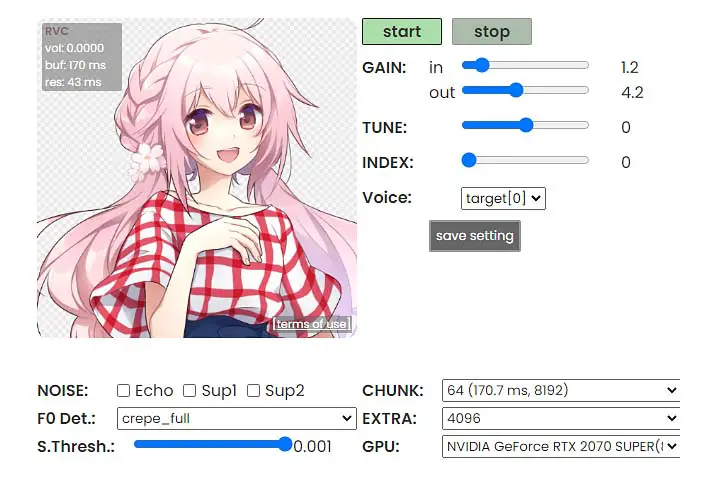
With AI software such as the Okada Live Voice Changer, RVC WebUI, or AICoverGen, which I’ve also ran on this very card while making tutorials for each of them, there really are no drawbacks connected with having less VRAM on board.
The inference is fast, the live voice conversion with the voice change is done almost instantaneously giving you only 200-500ms of latency on the audio output depending on your settings, and there really isn’t anything more to say here.
When it comes to software which doesn’t rely on loading large models into the GPUs video memory in their entirety, the 2070 Super performs really well. If you’re thinking of making some AI vocal covers, cloning character voices locally on your system or changing your voice live, you won’t be disappointed here. For these purposes, larger amounts of VRAM are simply not required.
If you’d like to go a step beyond however, and get into fine-tuning some RVC models, or tortoiseTTS/XTTS models used for voice cloning, you might find yourself needing more video memory depending on your preferred settings. It’s hard to hide the fact that 8GB VRAM GPUs in most cases really aren’t the most optimal choice if you’re really set on exploring different kinds of AI software and training or fine-tuning models locally on your system.
So In The End…
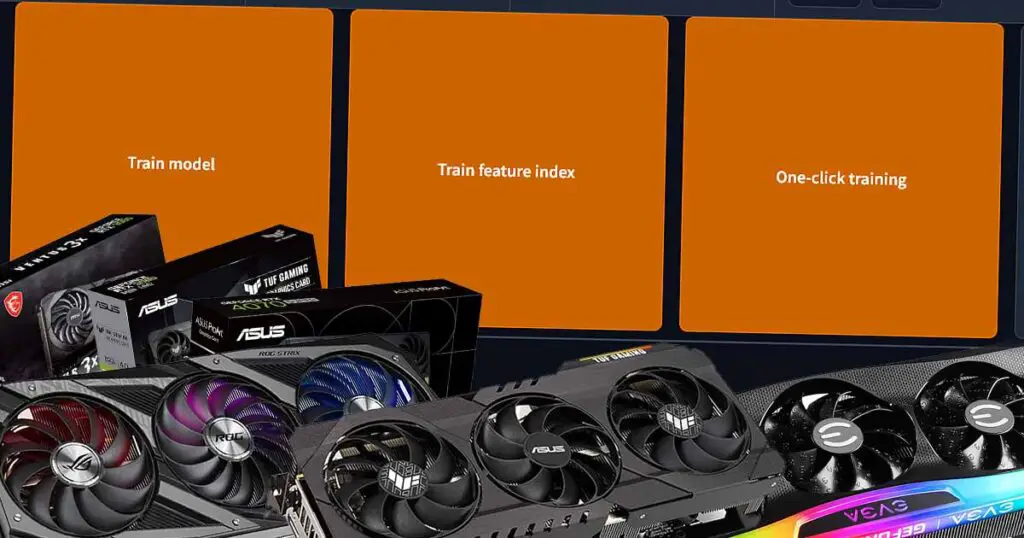
While I would certainly not recommend getting the RTX 2070 Super with local LLM hosting and AI model training/fine-tuning in mind, the card does perform really well when it comes to even the more recent games, lets you play some of them in 2k with stable 60-90 FPS with high graphical settings, and has no trouble with many local AI software which does not need to utilize more than 8GB of VRAM for loading up the models it requires to run.
So if you’re thinking of getting the 2070 Super specifically because you’re into AI, you’re most likely better off exploring some more recent options, because there are quite a few of them.
If however, you’ve found a deal that’s hard to turn down, or you already own this card, it will do more than enough for you if you bear in mind its hard limitations when it comes both to 2k gaming in higher framerates and low amount of VRAM which matters when locally hosting LLMs and training or fine-tuning larger AI models. I hope you found this helpful!