The dynamics in the consumer GPU market are slowly but surely changing. Just few months back I would be quite hesitant to recommend AMD graphics card to those of you who are just starting out with local AI including local LLMs. Now, many of the highly popular projects such as Ollama, LM Studio and OobaBooga WebUI are getting AMD GPU compatible versions and so, here is my new top list of the best cards you can get as of now, including some more budget friendly options. Let’s begin.
This web portal is reader-supported, and is a part of the Amazon Services LLC Associates Program and the eBay Partner Network. When you buy using links on our site, we may earn an affiliate commission!
How Much VRAM Do You Need?
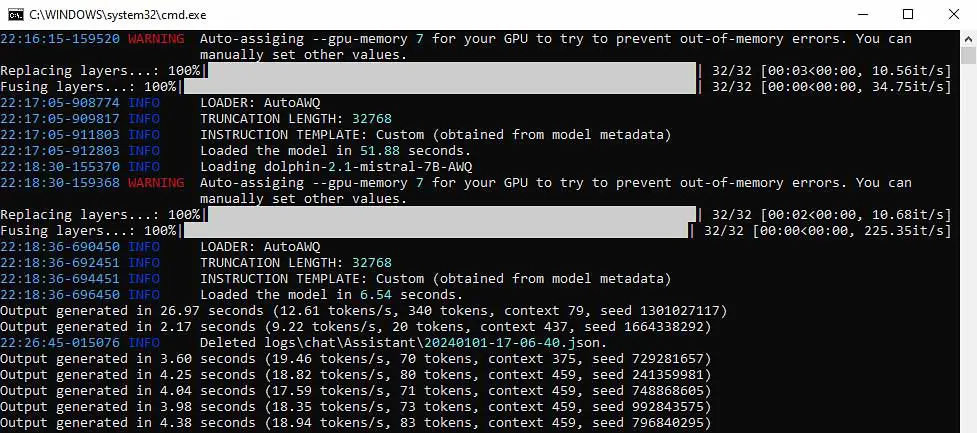
When setting up a local system for AI tasks like running large language models (LLMs), one factor should be the most important to you: the amount of video memory on your GPU (VRAM). For smooth and efficient LLM inference, having enough video memory is essential. The loaded model should, in an ideal situation, fit entirely within the GPU’s memory to avoid unwanted slowdowns caused by data being offloaded to system RAM. This is especially important when it comes to larger models, where high-quality results often demand more VRAM.
With that said, how much VRAM do you need? At this point in time, the maximum amount of VRAM you’ll find on a single consumer-grade GPU is 24GB, and that is what you should be going for if you’re serious about running larger, more complex models locally on your computer.
For smaller models, in general 12GB is considered the minimum recommended amount, and 8GB of VRAM isn’t really viable anymore if you’re buying a new graphics card for the purpose of utilizing local LLMs.
Should You Really Pick an AMD GPU?
While AMD GPUs can already run quite a few AI applications effectively and efficiently, they still fall a bit behind NVIDIA in terms of deep learning support due to their differences in utilized software frameworks. The Radeon Open Compute (ROCm) framework still does not offer the same level of compatibility or performance as NVIDIA’s CUDA technology, and among other reasons for that, one of the leading ones is the much higher popularity of the NVIDIA graphics cards over AMD ones in the current AI software market.
If you’re using software that primarily supports CUDA and doesn’t have AMD GPU compatible versions available, you might find yourself needing to necessitate some more or less complex workarounds or go through additional setup. It’s an important thing to keep in mind.
Still, many pieces of software widely used for locally hosting open-source large language models such as Ollama, LM Studio, Gpt4All and OobaBooga WebUI are fully compatible with AMD graphics cards and are pretty straightforward to use without an NVIDIA GPU. On the other hand, some local apps dealing with image generation using diffusion models are still behind when it comes to native AMD GPUs support, the ComfyUI being a good example here, at least for now. A good thing is that given the speed of advancements in various fields of AI in the recent years, the number of compatible software is likely to grow as time goes by.
Best AMD GPUs For Local LLMs and AI Software – The List
1. AMD Radeon RX 7900 XTX 24GB
If you’re serious about running high-end AI models locally using AMD hardware and you have the money to spare, the Radeon RX 7900 XTX definitely is the best card on this list.
With 24GB of GDDR6 VRAM, it’s directly comparable to the NVIDIA RTX 4080 and 4090 being capable of loading many of the larger models without without needing to offload data to your system memory. This card is ideal for running more complex LLMs with higher precision or minimal quantization, which as you might already know, should be your end goal.
NVIDIA Equivalent: GeForce RTX 4080 16GB / GeForce RTX 4090 24GB.
2. AMD Radeon RX 7900 XT 20GB
Just slightly below the XTX, the AMD Radeon RX 7900 XT still packs an impressive 20GB of VRAM, which is noticeably more (4GB more to be exact) than its NVIDIA equivalent – the RTX 4070 Ti.
While it’s a visible step down from 24GB of VRAM, it’s still enough to handle most larger LLMs efficiently, especially when using models with higher level of quantization. If your budget doesn’t allow for the top-tier 7900 XTX, this is also a great option for advanced AI tasks.
NVIDIA Equivalent: GeForce RTX 4070 Ti 16GB
3. AMD Radeon RX 6800 XT 16GB
For users working with slightly smaller LLMs or moderate quantization levels, the AMD Radeon RX 6800 XT is an excellent middle-ground choice, coming right after the 7900 XT.
Its 16GB of VRAM is more than enough for running multiple 7B models and 11B models in 4-bit quantization, and it still gives you quite a lot of headroom. While it won’t handle the largest models with the same efficiency as the 7900 XTX, it’s still a solid performer for most local AI setups. In terms of performance, it’s closest NVIDIA counterpart seems to be the RTX 3080 10GB, or the RTX 4060 Ti 16GB.
NVIDIA Equivalent: GeForce RTX 4060 Ti 16GB
4. AMD Radeon RX 6800 16GB
There is also a non-XT variant of the previously mentioned card that’s still worth looking at. The base Radeon RX 6800, is another neat proposition from AMD.
This card also offers 16GB of VRAM, making it an event more cost-effective choice for all of you who need a solid amount of memory but don’t require top-end performance, which in most cases is a totally viable approach when talking about locally hosting smaller large language models. A pretty good choice overall, and for an even better price.
NVIDIA Equivalent: GeForce RTX 3070 8GB / GeForce RTX 3080 10GB (not really worth it with the relatively smaller amounts of VRAM they offer)
5. AMD Radeon RX 6700 XT 12GB
The AMD Radeon RX 6700 XT 12GB alongside a slightly more powerful RX 6750 XT 12GB are yet another great entry-level options, this time even more affordable.
With 12GB of VRAM, this card can still handle 7B and 11B models without any trouble. It’s the minimum recommended VRAM for some more serious LLMs work, making it a decent choice if you’re working with simpler tasks, smaller content windows, or models with lower parameter counts. This is also the least expensive card on this list, without getting into the 8GB VRAM GPUs, which while they certainly can let you utilize smaller 7B models in 4-bit quantization with lower content window values, are far from being “the best” for local AI enthusiasts.
NVIDIA Equivalent: GeForce RTX 3060 12GB
6. AMD Radeon PRO W6800 32GB
This one is a little bit different. If you’re looking for an AMD card with significantly more video memory than the 7900 XTX (and the NVIDIA RTX 4090), the Radeon PRO W6800 has an astounding 32GB of GDDR6 VRAM on board.
This card is quite obviously marketed more towards professional users. Its massive 32GB VRAM capacity makes it perfect for handling extremely large models and datasets, and can make fine-tuning larger models using LoRAs much easier. If your workflow involves high-precision models, heavy multitasking, or managing massive datasets, this card will deliver the memory and performance needed. Make sure that your motherboard and power supply are compatible with this card before getting it though!
NVIDIA Equivalent: NVIDIA RTX A6000 48GB (A word of warning: this one, although it does have even more video memory, is at the same time almost 4x more expensive)
So, How To Choose? – Which One Should You Pick Up?
When it comes to local LLM inference, one rule is generally true: the more VRAM, the better. When dealing with larger models and larger context windows, more video memory can allow for faster and more efficient model inference by avoiding system memory (RAM) offloading, which can significantly slow down your operations.
- For those working with advanced models and high-precision data, 24GB VRAM cards like the RX 7900 XTX are the best bet, and with the right setup and enough money, you could even go for the Radeon PRO W6800 32GB if you feel the need for quick model switching, easier LLM LoRA training, or simply loading larger, higher quality models in higher precision.
- If you can settle with loading smaller quantized models but still require significant computing power, the RX 7900 XT and RX 6800 XT are both very solid choices.
- On a budget? The RX 6700 XT provides decent performance for smaller models and tasks.
In the very end your choice should depend on your specific workload, the size of models and the type of software you plan to use, and of course, your budget. While AMD may still lag a little bit behind NVIDIA in the space of AI applications, the cards above are still great picks when you’re using them with compatible software. Here you can find a similar list, but featuring cards from NVIDIA. Hope you found what you were looking for!